If you've been working in the data science field for any length of time, you know there are two dominant languages that are used for this purpose: R and Python. The choice between the two has been largely a matter of preference. Both will suffice to give you the tools you need to get a majority of your data science work complete.
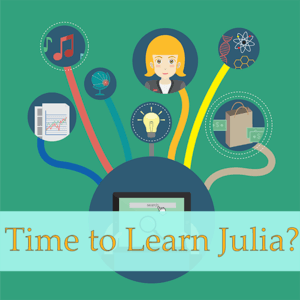
If you are new to programming, you will likely read that Python is an easier language to learn. As a computer science major in college and one who has worked in the field for over 22+ years, I concur. Python is easier to learn.
However, once you get cracking with R, and if you choose this language from the start, you'll see why it has a cult-like following. It just makes a lot sense. I can't remember the last time I had to use a loop in this language. Just saying... R coders will know what I am talking about.
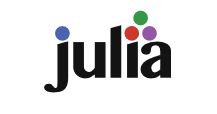
Julia is jumping on that bandwagon too, according to the author. If this trend continues, you won't really have to worry about choosing the wrong language as you can write in whichever one you have been working with. This is good news for the most part. If there is a library that does something one of the three, the interoperability factor will let you have your cake and eat it too.
What Mr. Miller wrote about should be considered a positive development overall. However, as the owner of a review website dedicated to data science, it now looks like I am going to have to learn the Julia language after all. How can I review something I don't know about? Ah well! That is my cross to bear! I'll write a post here about my experience when I get around to learning Julia.
It's all good!