Data science is an exciting field but has its share of challenges. One of those challenges is the demand for computing power continues to grow. Data science practitioners and enthusiasts are concerned they will be “powered out” of the field. Are machine learning algorithms and artificial intelligence now only useful to big corporations? There is hope for smaller players and this article will help you see that it’s time for data scientists to get their heads into the cloud.
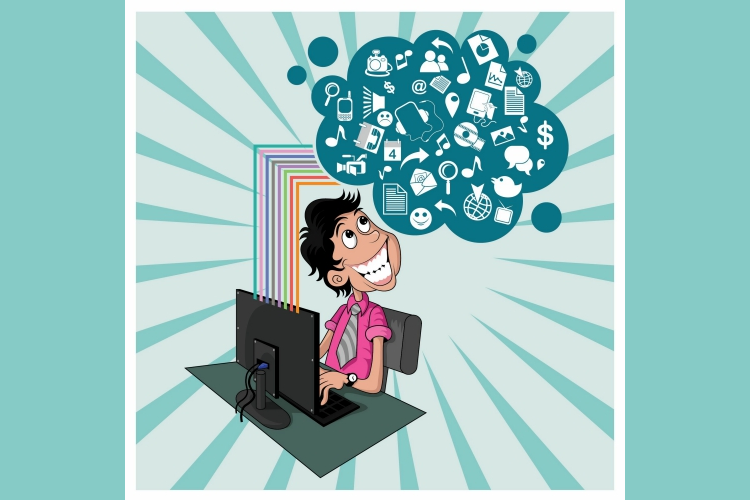
By now, most small business owners have learned that they must use their data to gain a competitive edge. But what does that even mean for most of these businesses? Some people are adept at using spreadsheets, but that skill alone is not enough to perform data analytics. It may handle some of the jobs, but for most, spreadsheets fall short.
Artificial intelligence (AI) is growing in popularity and large corporations are jumping on the bandwagon. This development puts them at a huge advantage over their small- to medium- business (SMB) competitors.
AI is a computing-resource hog. Even machine learning algorithms (a subset of AI) show growing needs for more computing power. This power obviously comes at a cost, one which most SMBs can’t afford.
But small business owners are not the only group impacted by the growing demands in computing power. Many freelance data scientists are feeling the impact. They too, don’t have the resources to beef up their computing power, nor do many of the smaller companies they work for.
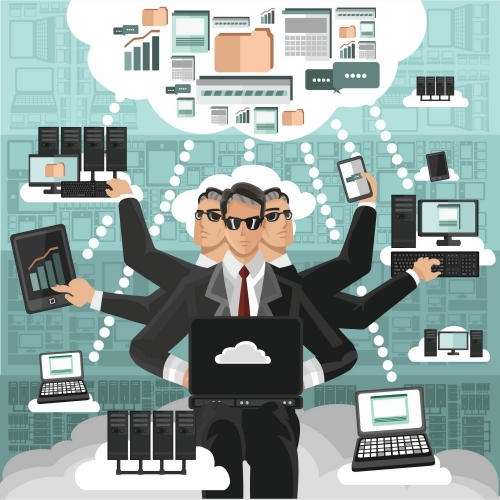
Luckily, cloud computing has provided the means for all companies and data scientists to take advantage of high-end computing resources. Before the cloud, data scientists would need to spend thousands of dollars on computers to run machine learning algorithms.
Technology also has a way of becoming obsolete quickly. A machine that had a top configuration two years ago, is likely to be ineffective today.
The growth of data is another challenge. AI algorithms tend to increase accuracy with more data fed to them. Large corporations are pouring billions of dollars into data centers and more powerful processors.
Cloud providers are giving SMBs and data scientists a chance to stay in the game. These providers invest heavily in their infrastructures and computing power. Many are even gearing their services for machine learning.
Cloud computing can answer the increased demand for computing power and the growing need to process massive amounts of data.
What About the Costs of Cloud Computing?
Cloud computing does cost money and the amount depends on how companies use the services. For some services, they charge for the amount of resources you use. For others, you may be billed for selecting those options, irrespective of usage. You may also be charged for bandwidth or throughput.
Due to these charging schemes, it’s vital for data scientists and companies to understand what they will be paying. Otherwise, you can be hit with unexpected surcharges. These charges can be significant, too.
The good news is that the major cloud service providers have pricing calculators and other cost estimation tools to help. Still, you’ll need to understand which services (Etro, 2012) make sense for your situation. This takes practice and learning.
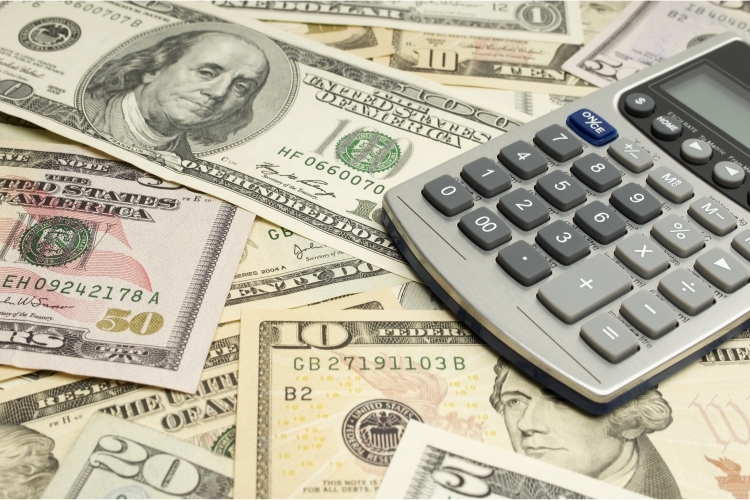
Some may find these constraints off-putting. However, the benefits are likely to outweigh these constraints. What would it take for data scientists to put together the same hardware, software, and networking requirements that are available in the cloud?
When looked at in that light, data scientists should view cloud solutions as a way to save money. If nothing else, it enables them to run their models on computing power that is normally beyond their reach.
Learning about cloud computing is often a matter of doing it. Amazon, Microsoft, and Google all allow users to sign up free and use a subset of the services for free. This often includes computing, storage, and machine learning algorithms. You will have to monitor your use of these services since if you go over the allotted amount, you will be charged.
It does provide you a low-cost way to learn about the cloud and what is available. If you do incur any costs while learning, think of it as part of your tuition. Or you can chalk it up to spending money on a proof-of-concept.
Upgrades Available
Before cloud computing was available, tech people had to guess at how much computing power was needed. They would often account for more power than was needed to ensure they didn’t fall short when demand increased. With cloud computing, you can scale up as your demands increase.
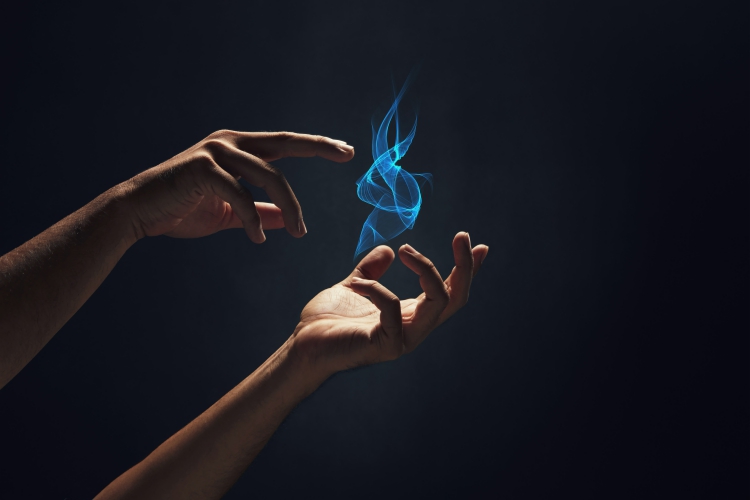
Subscribers to cloud providers get access to any upgrades in technology. Unless there are reasons to limit the upgrades for members, each member can request the new technology and it will be added to their service.
The upside to cloud providers is you never have to worry about running out of computing power. Of course, the cloud providers may charge more for the upgrades, but that’s not always the case. Sometimes, to keep from becoming obsolete, old servers are retired and the newer technologies take over. In this case, it is a status quo scenario and the provider may not charge more for this replacement.
Like Your Configuration? Create an Image of a Typical Config
With Amazon’s AWS, members can create and load Amazon Machine Images (AMI). Suppose you have a configuration that works well with your data science projects. You can save the configuration to an AMI and anyone who needs access to this configuration can load up the image.
If you have multiple configurations that you use for your data science work, you can save each as a custom image and load them as you need them. Also, you can have multiple instances with the different configurations already loaded.
The Primary Cloud Providers Cater to Machine Learning
Amazon, Google, and Microsoft are all including machine learning and AI features as part of their service offerings. This trend does not seem to be slowing down anytime soon. They also provide pipelines to help you manage your machine learning workflows.
You are not required to use their AI services. You can set up your virtual machines with software used by your company. However, if the services offered provide everything you need for your job, you can continue to use those options.
Conclusion
Data scientists often complain that much of their time is spent on tinkering with configurations. The rising demand for computing power makes it even more difficult to manage without help. Small businesses don’t have the resources to provide that help. Cloud providers offer managed services that offload a good portion of the mundane tasks that data scientists find themselves doing day-to-day. Cloud technologies have a learning curve, but it is not insurmountable. Once learned, it opens up a world of possibilities.
Bibliography
Etro, F. (2012). The Economics of Cloud Computing. The IUP Journal of Managerial Economics(2), 7-22. Retrieved 10 6, 2020, from https://igi-global.com/chapter/the-economics-of-cloud-computing/119952